Multi-Echelon Inventory Optimization Software
Inventory Optimization for the Real World
More Realistic Safety Stock Recommendations and Easier to Use
Many multi-echelon inventory optimization (MEIO) solutions produce unrealistic recommendations and are difficult to use. New Horizon MEIO takes a different approach and produces more realistic safety stock plans using a more intuitive user experience.
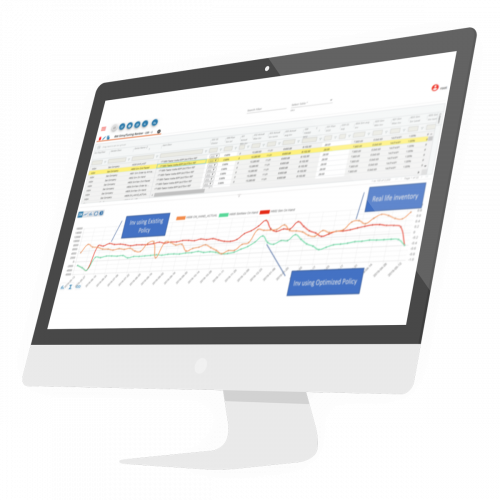
Why Choose Our Multi-Echelon Inventory Optimization Software?
Produce More Realistic Recommendations Using Machine Learning Algorithms
Other MEIO solutions assume a normal distribution of error. New Horizon makes no such assumption. We apply machine learning to your historical inventory and service level data to produce realistic safety stock recommendations you can plan on.
Increase Accuracy by Tailoring Recommendations to the Forecasting Algorithm
Inventory optimization must take into account demand variability and thus how the demand plan was generated. To produce more accurate results, New Horizon tailors its calculations to the specific forecasting algorithm used to create the demand plan.
Focus on Key Items Using Exception Management
New Horizon MEIO identifies a watch list of problematic and opportunity items so that users can focus on them during the forecasting and procurement processes. Automate the management of routine items so you can focus on what’s important.
Proactively Address Potential Problems Using What-If Capabilities
Simulate what will happen using different safety stock policies so today’s recommendations don’t cause a problem in the future. Identify potential issues before they happen.
Maximize Adoption and Productivity with an Intuitive User Experience
New Horizon’s user experience (UX) is based on lessons learned from over 100 planning implementations. Our modern UX is intuitive and includes features such as highly configurable layouts and easy identification of exceptions.
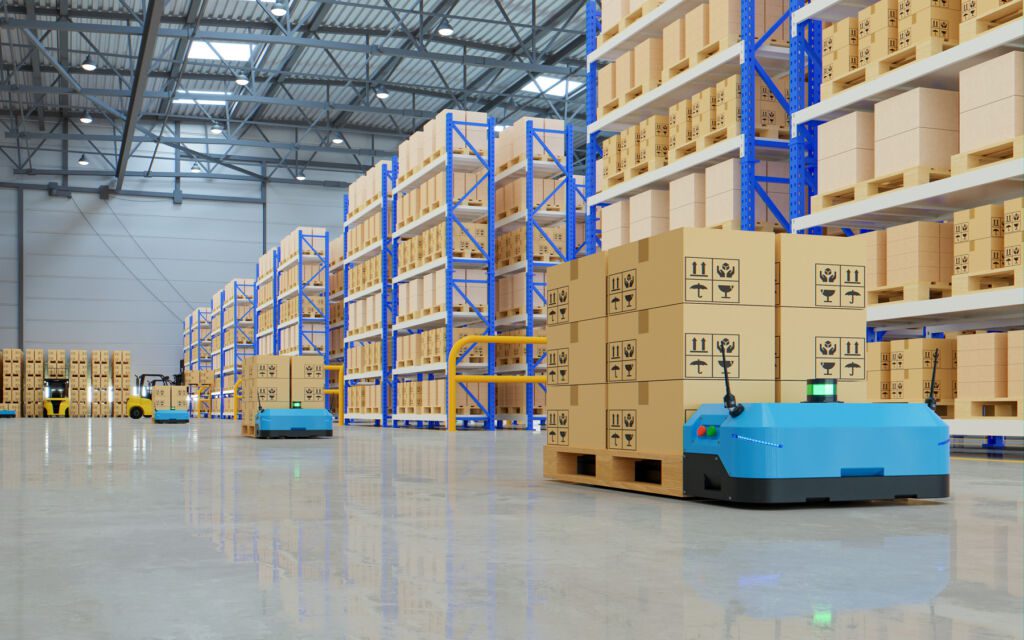
Advanced Features for Multi-Echelon Inventory Optimization
- Manage inventory holistically. Take a multi-echelon approach to distribute inventory optimally across different network nodes, not at each node in isolation.
- Use AI to continuously improve safety stock policies. Adjust recommendations based on historical performance.
- Control total inventory costs. Optimally allocate safety stock subject to a total network-wide inventory investment constraint.
- Measure potential results. Analyze historic data to assess expected improvement in service level prior to deploying solution.
“New Horizon’s MEIO product is a new generation solution which creates realistic recommendations for safety stock levels.”
Director of Inventory Management
Wholesale Distributor
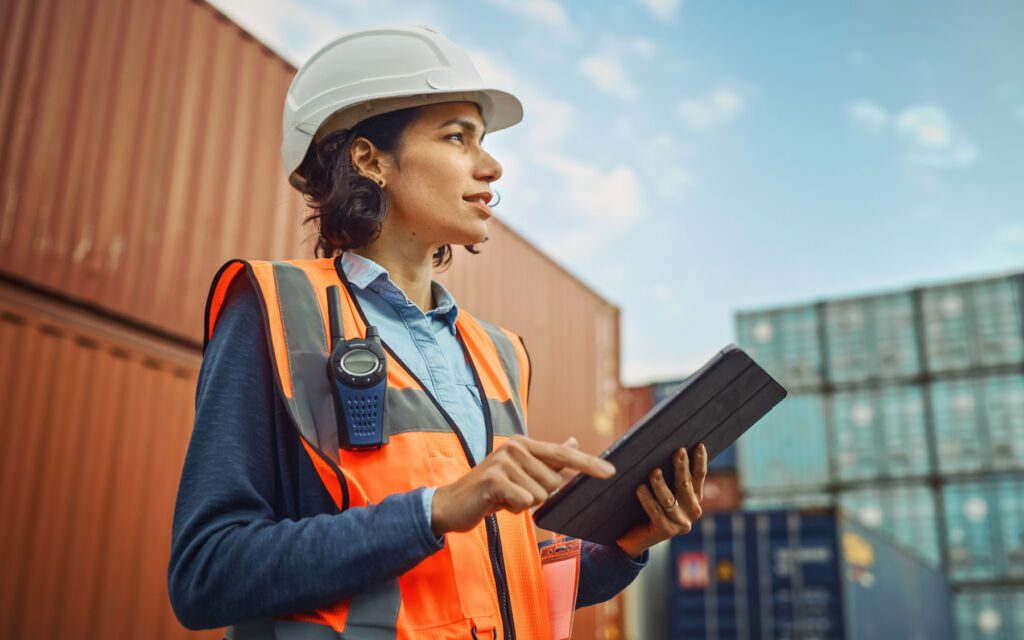
Create Optimal Multi-Echelon Inventory Plans
Multi-Echelon Inventory Optimization uses the power of your data to take inventory management to a new level. With MEIO, you can scientifically set optimal safety stock levels in a multi-tier distribution network. Benefits include:
Up to 33% inventory reduction while maintaining product availability
Ability to consistently meet target customer service levels
Increase in revenue resulting from improved service levels
Reduction in spoilage for perishables and price erosion for short product lifecycle items
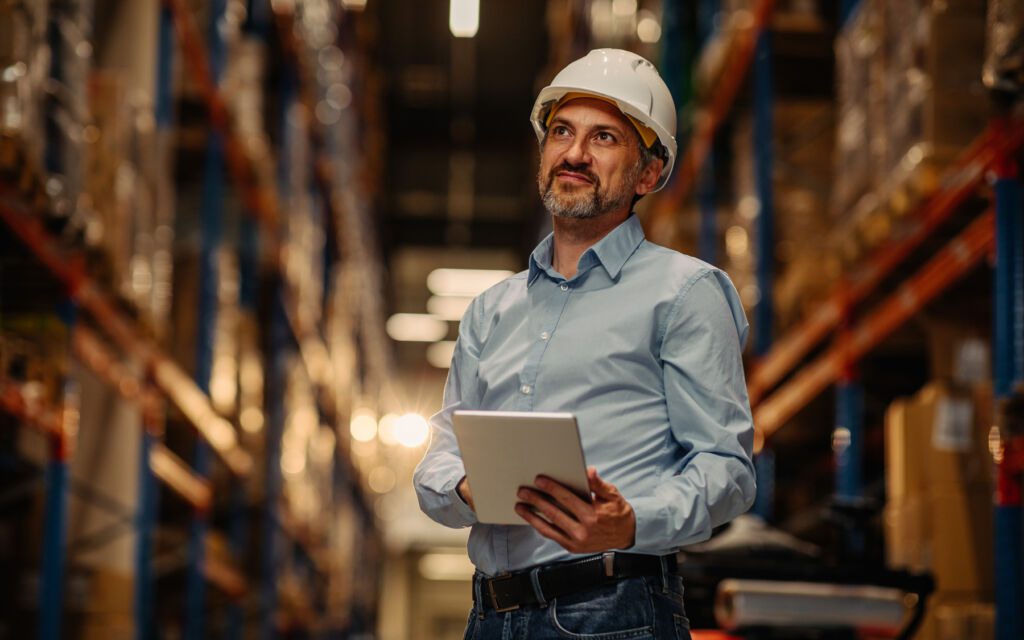
Built on an Enterprise-Class Supply Chain Platform
Multi-Echelon Inventory Optimization is built on the New Horizon Platform, which provides a more intelligent yet simpler planning solution by taking full advantage of the latest cloud and data science technologies. The result is an intuitive user experience, faster time to value, and better plans. Planning Made Easy™.